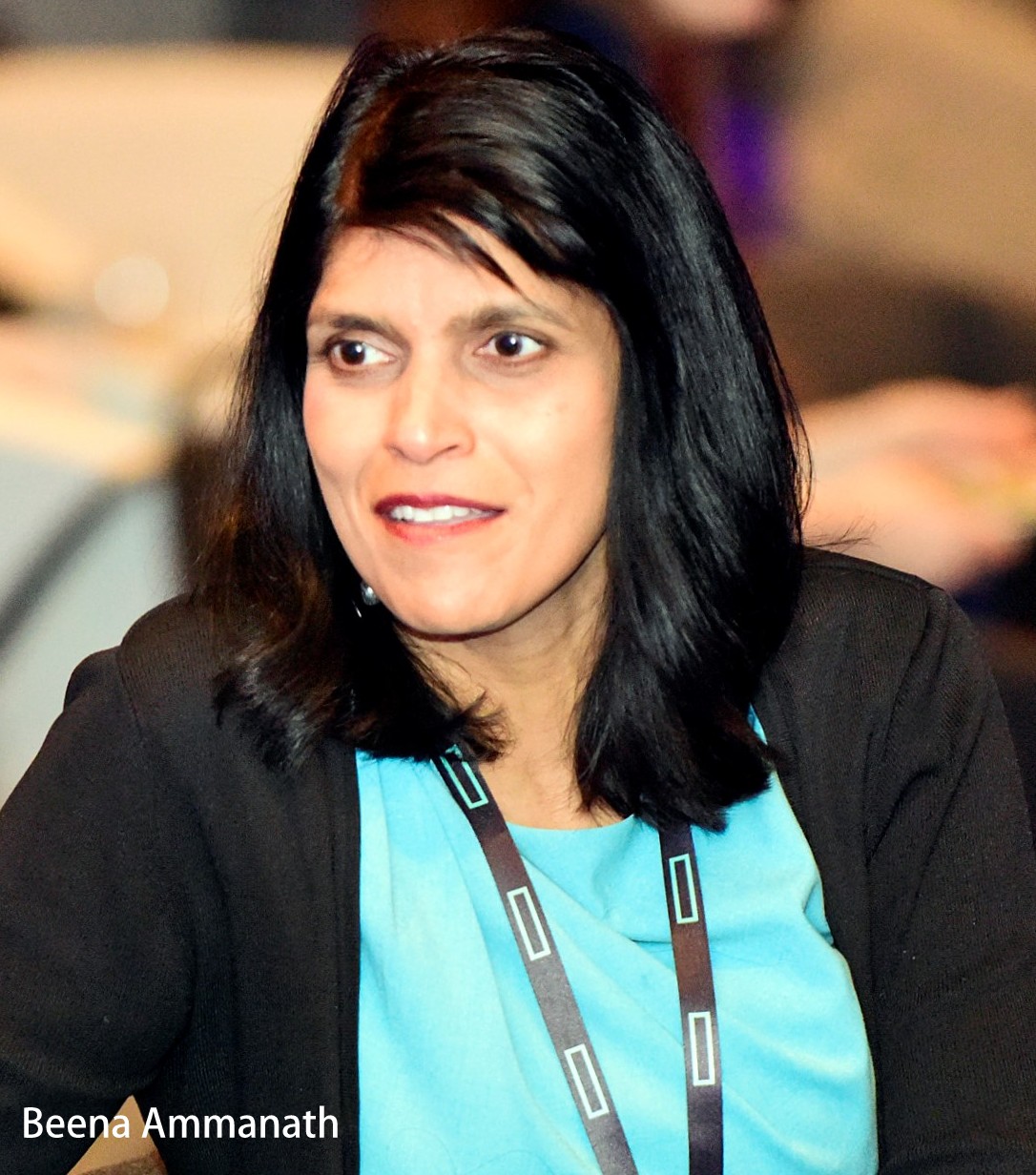
Manufacturing has been continuously evolving since the first Industrial Revolution. Early manufacturing was often done in people’s homes, using hand tools or basic machines. The First Industrial Revolution used steam power to mechanize production. The Second used electric power to create mass production. The Third used electronics and information technology to automate production and this still continues. Now we are again at the brink of a change. The digital revolution is happening as the manufacturing factory floor gets connected and we are able to achieve production at a scale with lower cost and increased quality. Changing consumer demand and market dynamics such as increasing energy, labor costs, flexibility needs put pressure on manufacturing companies to become smarter in connecting business processes, operational, IT data, people, and things.
As the cost of the sensors, data storage, high-performance compute and analytical engines are coming down and with increasing stream of data from thousands of devices, it is no longer possible for specialists to analyze and contextualize all this environmental data in a short time-frame. Machine learning, vision, and other fields of AI emerge to meet these rising demands and transform the way people and machines work together.
Artificial Intelligence is here to stay for the next decade and beyond bringing revolutionary changes to the manufacturing and high-tech industry. Organizations should understand their business needs first and find the best use cases to apply AI.
Imagine how maintenance of manufacturing assets change based on the real-time analytics of variety and volumes of data coming from Internet of Things (IoT). Machine-learning provides the way to analyze the past and future patterns of the data and prescribes the best possible actions significantly enhancing the decision making process. Automating the maintenance actions based on insights become possible.
While predictive analytics tells us why an equipment is likely to fail the objective of prescriptive maintenance is to optimize the outcome based on prediction. As example, using AI techniques like Gradient Tree Boosting it can be predicted that an equipment is going to fail with a probability of more than 90% with concrete prediction and failure timeframes. In addition to this, contextual business data, failure impact, cost of proactive fix are also analyzed. This not only opens the ‘prediction black-box’ but provides hints about actions to consider. The next step is to manually or automatically link the prediction to a pre-defined action, generating a maintenance ticket or even shutdown the equipment.
For example, a steel company needed to stop the production line in case of defect. That took a long time delaying the production. Working with HPE Pointnext, services experts of Hewlett Packard Enterprise (HPE), the steel manufacturer managed to predict maintenance 1 month before the failure, eliminating unneeded expensive post -production control, preventing production defects and reducing the number of downtimes. In another project, an aluminum maker was looking to improve the quality management of the aluminum anodes. Any malfunctioning anode, weather it cracks or falls apart, increases the consumption of electricity, reduces the quality of the final aluminum and requires a team of experts to deal with the situation. Working with HPE Pointnext, the company implemented a predictive model to identify the anode blocks that are likely to fail in production before they are actually used. This increased energy efficiency and the quality of the final product.
Original equipment and IT system providers of manufacturers are also embedding AI and machine learning addressing specific use cases. For example HPE uses machine learning in its storage solutions in two ways.
Firstly, with the supervised learning , the storage (HPE Nimble Storage in this case) learns what causes bad outcomes and can start to understand what may have happened should a bad outcome occur. In order to do this, Nimble Storage doesn’t just look at logs and events from storage devices. It must gather other data from the infrastructure too. Secondly, Nimble Storage uses the same techniques to proactively determine that customers are running out of storage capacity. This is really a form of proactive maintenance, where the “maintenance fix” is to add more storage.
Manufacturing similar to Energy & utilities, aerospace & defense and transportation & logistics industries largely depend on efficient and smooth operations with specialized equipment and devices. Prescriptive Maintenance come-up as one of the top uses cases taking advantage of machine learning and AI. Leading manufacturers will anticipate what might or will happen to their assets by exploring, experimenting and then scaling each of the use cases starting with a low hanging fruit like prescriptive maintenance.